Keynote presentation at the English Australia Conference, Perth, Australia, 11–13 September 2024
Presentation
I would like to begin by acknowledging that teaching and learning have been taking place for thousands of years right here on the traditional lands of the Noongar people, in what we now call Perth, Western Australia. I would also like to recognise that we can learn a lot from past teaching practices that can help to inform our future teaching practices.
1. Introduction: Not another revolution!
My presentation today focuses on generative artificial intelligence (AI), which some people claim is about to revolutionise education. We will start by zooming out to take a technological view, which is relevant to education in general; next we will zoom in to take an educational and assessment view, with examples selected to specifically illustrate language teaching and learning wherever possible; and then we will zoom out once more to take a societal view, which is again relevant to all areas of education. Finally, we will wrap up with some brief comments on AI literacy.
First, however, I would like to say a few words about revolutions. The term ‘revolution’ can refer to the forcible, often violent, overthrow of an outdated system in favour of a new one. The term can also refer more generically to the complete, radical overhaul of an old system. For more than a century, enthusiasts have been promising us an educational technology revolution. Radios were going to allow widely accessible, up-to-date, home-delivered learning; so, too, were televisions. Computers were going to allow all of the above, along with collaborative networking; so, too, were mobile phones. If we ask ourselves whether these devices have improved education, the answer is: absolutely. But if we ask ourselves whether these devices have revolutionised education, the answer must be: far from it. We still have ministries and departments of education, schools and universities, curricula and textbooks, teachers and classrooms, students and assignments. What these devices have done is not to support an educational revolution, but rather to support educational evolution, with the latter term understood to mean a slow, stage-by-stage development.
Undaunted by the failure of past edtech revolutions to materialise, enthusiasts now promise us that the AI revolution is coming for education. The image shown here (see Fig. 1) is a composite of key features of the imagery typically accompanying such predictions: a robot teacher; rows of empty desks, with students either absent or online; and classroom décor which seems to be modelled on schools of a century ago, complete with chalkboards (indeed, chalkboards seem to be everywhere in these images).

Figure 1. A humanoid robot teaching. DALL-E 3 image created by Mark Pegrum (2024) via Microsoft Copilot.
While this image is a composite, let us now look at the text of some actual titles, headlines, and slogans from readily accessible online sources – aligned sometimes closely and sometimes more loosely with the fields of education and technology, and sometimes with a more or less commercial bent – as typified by the very generic: ‘Artificial intelligence for education revolution’ (Gurung, Reference Gurung2022). For educators who work in a school, AI will be ‘[r]evolutionizing K–12 education’ (Lim, Reference Lim2023); for those who work in or attend a university, AI will be ‘revolutionizing higher education’ (ASM Research Publications, 2023); while those who work with international students, as many language teachers do, can look forward to the ‘revolution of international education’ (Focus Education, 2024). Implying that contemporary education is effectively a problem in search of a solution, another source promises, with somewhat unusual phraseology, ‘AI solutions for education industry that can bring revolution’ (Matellio, 2022).
So what exactly might this revolution be expected to entail? As exemplified by the title ‘Learn faster and better with AI’ (Learnboost, n.d.), there is some excitement about acceleration, based on the dubious assumption that rapid learning is automatically better than more gradual learning. However, the greatest enthusiasm is reserved for predictions about the growing ‘personalisation’ of learning; to cite just two recent instances: ‘Education revolution: The rise of the AI-powered personalised classroom’ (Thompson, Reference Thompson2023) and ‘Transforming education: AI-powered personalized learning revolution’ (Zia, Reference Zia2023). This is highly ironic in the context of accompanying images populated by robots and screens, without a single person in sight. This is a point to which we will return.
Without fail, what these articles and posts promise us, as teachers or students or both, is help in ‘[n]avigating the AI revolution in education’ (Picardo, Reference Picardo2023). This may be just as well, because otherwise – to conclude with a mildly threatening headline – ‘[t]he AI reeducation revolution is coming for you!’ (West, Reference West2023).
Why, then, do I not share the view that generative AI, or any other educational technology, is going to revolutionise education? It is essentially because, as Justin Reich points out in his 2020 book, Failure to disrupt, education is a complex ecosystem which serves multiple purposes and multiple stakeholders; these include students, teachers, parents, employers, and governments. Technology is just one element within this large ecosystem. Observing that acceleration may not be an unadulterated good in education, Reich notes: ‘Trying to accelerate learning by ramping up technology is like putting rockets on butterfly wings. More force does not lead linearly to more progress’ (Reich, Reference Reich2020, p. 9). He later elaborates:
Improvements in education very rarely, perhaps never, come by way of dramatic transformations. They come through deep, long-term commitment to the plodding work of building more robust systems. … New technologies get introduced into complex learning ecologies, and those complex learning ecologies require multiple changes at multiple levels to take advantage of new technologies. … changes in educational systems are necessarily incremental, but step change is what continuous, incremental change looks like from a distance. (pp. 243 and 245)
In other words, it is through slow, careful stages that we arrive at step change, or major change, or major forms of evolution in education.
Echoing this point from a practical rather than a theoretical perspective, the British Council’s 2023 report on Artificial Intelligence and English language teaching, based on a survey of over 1300 teachers and interviews with almost 20 edtech experts, presents a picture of ‘the future as one of continuity rather than widespread disruption of educational systems’ (Edmett et al., Reference Edmett, Ichaporia, Crompton and Crichton2023, p. 50).
In my view, therefore, it is time to stop talking about an AI revolution in education, and instead to start talking about how AI can helpfully support the evolution of education in areas where change might be needed. Leaving behind the distractions of revolutionary rhetoric, this presentation will, as indicated, focus on the present and likely future technological aspects of AI, before turning to its educational and assessment implications, and then its broader societal challenges. As we undertake this journey, we should bear in mind that AI could help to make education better in some ways, but it could also make education worse in other ways. Consequently, as educators, we need to develop the AI literacy to ensure that AI is supporting the evolution of education in what we believe to be a positive direction.
2. A technological view
Generative AI first came to wide public attention with the release of ChatGPT in late 2022. It became the fastest digital service ever to reach one million users, which occurred within five days of its release (though it was surpassed the following year by Threads, which arguably benefited from its link with Instagram; Buchholz, Reference Buchholz2023). Let us take a step back for a moment to explore how ChatGPT fits into the bigger picture of artificial intelligence.
You may be aware that the broad field of AI can be roughly divided into two subfields (Pegrum, Reference Pegrum2019). Strong AI, or general AI, refers to the ability of machines to apply intelligence to a whole range of tasks, much as humans do. Despite many decades of research, strong AI remains for now largely the province of utopian, or perhaps more often, dystopian science fiction. Think of 2001: A Space Odyssey (1968), Westworld (1973), Blade Runner (1982), The Terminator (1984), The Matrix (1999), Her (2013), Ex Machina (2014), I Am Mother (2019), or Foundation (2021 onwards); this has been a long-standing cinematic obsession.
By contrast, weak AI, or narrow AI, refers to the ability of machines to apply intelligence to very specific tasks, which generally involve very specific forms of pattern matching, and where these machines can frequently exceed human abilities. Recent years have seen enormous advances in this area, thanks to machine learning and, in particular, deep learning based on artificial neural networks. Some of the outcomes are seen in today’s image and object recognition, voice recognition, natural language processing, automated translation, and learning analytics. Thus, it can be said that for now the broad field of AI is absolutely dominated by the subfield of weak AI.
Within this subfield of weak AI, we find both conversational and generative AI. Conversational AI is trained on huge datasets of human conversations, and its function is to mimic such conversations. Conversational agents, with which humans can interact directly, include organisational or corporate chatbots, and first-generation voice assistants like Amazon’s Alexa or Apple’s Siri. By contrast, generative AI is grounded in large language models (LLMs) and trained on vast datasets of texts and other artefacts. Its function is to generate new, or at least remixed, content, in response to human prompts. Examples include GPT.
The major breakthrough made by the San Francisco-based company OpenAI, as released to the public on 30 November 2022, involved combining generative AI in the form of GPT with the ethos of conversational AI in the form of a chat interface, resulting in ChatGPT. It was this combination, and this ability to chat with generative AI, which really captured the public’s imagination and which has led to the subsequent proliferation of generative AI tools.
Generative AI does not only involve text generation. One of the best-known of today’s image generators is DALL-E, also from OpenAI, which produces images in response to text prompts; other examples include Adobe’s Firefly, Midjourney, and Stability AI’s Stable Diffusion. To illustrate how fast this technology is advancing, compare the image I produced for my website in 2023 with DALL-E 2, using the prompt ‘the future development of web 3.0 and artificial intelligence, in the style of digital art’, with the image I produced in 2024 with the next-generation DALL-E 3, using exactly the same prompt (see Fig. 2).
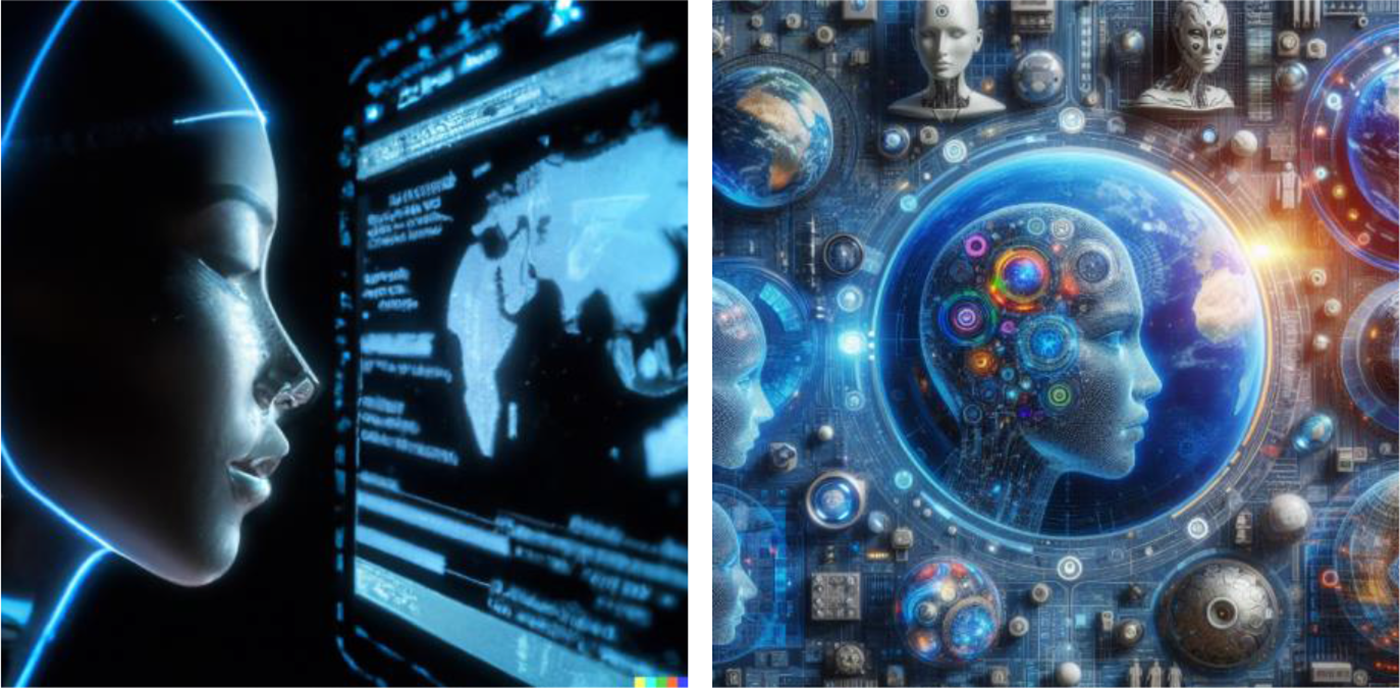
Figure 2. Future of web 3.0 & AI. On left: DALL-E 2 image created by Mark Pegrum (2023) via Microsoft Copilot. On right: DALL-E 3 image created by Mark Pegrum (2024) via Microsoft Copilot.
Audio and video are increasingly well-represented among generative AI tools. For instance, Microsoft’s VALL-E, given a 3-second recording of a person speaking, can create an audio file simulating the same voice speaking whatever text a user inputs, while VALL-E X can then render it in multiple languages (note that these tools are not currently available to the public). The second half of 2024 has seen the arrival of an array of highly sophisticated video generation software, including Luma Labs’ Dream Machine, OpenAI’s Sora, Runway’s Gen-3, and Kuaishou’s Kling AI, all of it readily available to everyday end users.
Before returning to ChatGPT itself, I would like to comment briefly on the use of terminology. We often talk about technology in metaphorical terms; all metaphors reveal certain things, but also hide certain things. I am sure you have heard the term hallucinations used to refer to the incorrect or simply invented information sometimes generated by AI in response to human queries. What is important to understand here is that generative AI is just probabilistic pattern-matching software. It predicts the next word – or the next pixel or the next note – based on the patterns it identifies in its datasets. To put it another way, LLMs are in essence ‘incredibly sophisticated autocomplete systems’ (Mollick, Reference Mollick2024b). Generative AI has no comprehension of texts or other media, much less of the external world to which they refer. From another angle, then, we might say that everything produced by generative AI is actually a hallucination (cf. Franks, Reference Franks2023). It is fortunate for us that most of the time these hallucinations (grounded as they are in human datasets) do in fact correspond to our understandings and our perceptions of the external world.
Then there is the term generative AI itself. Perhaps a better term, as suggested by Massachusetts Institute of Technology (MIT) Media Lab computer scientist Joy Buolamwini, might be regurgitative AI (Brown, Reference Brown2024). What is important to understand here is that current AI is incapable of creating, or generating, anything new. All it can do is to remix, at a very granular level, existing content originally created by human beings. As for whether and to what extent a remix can be considered original, that is a question we will leave for another day.
Let us now turn to GPT, the LLM that underpins ChatGPT. Existing since 2018 in evolving iterations, GPT has been used for some time across a range of industries, for example to generate code, to write news articles, and to support the production of marketing materials. When ChatGPT was first released, it was based on GPT-3.5.
To interact with a generative AI chatbot like ChatGPT, we can start by inputting a text-based or voice-based question or command, known as a prompt. It is vital to use the right prompt to get the right output, so much so that we are now seeing the emergence of a new field, and indeed a new profession, which is being referred to as prompt engineering:
Like writing and coding before it, prompt engineering is an emergent form of thinking. It lies somewhere between conversation and query, between programming and prose. It is the one part of this fast-changing, uncertain future that feels distinctly human. (Warzel, Reference Warzel2023)
Interestingly, in 2023, Matt Bell from Anthropic, the company behind the generative AI chatbot Claude, told an interviewer: ‘In fact, our best prompter is a philosopher. Good prompting involves writing extremely clear explanations … and figuring out reasons for misunderstandings and how to prevent them’ (cited in Purtill, Reference Purtill2023). For many decades, educators have been supporting students in developing search literacy, encompassing the ability to compose carefully considered and appropriately detailed search strings in order to access the desired search results. What we might call prompt literacy (Gattupalli et al., Reference Gattupalli, Maloy and Edwards2023; Hwang et al., Reference Hwang, Lee and Shin2023) is really an extension of this idea. While there may be a small number of specialised – and highly lucrative – job openings for prompt engineers in the future, all users of generative AI will need to develop a certain degree of prompt literacy to support effective everyday interactions with this technology.
While ChatGPT and its underlying LLM are continuing to evolve, there are also numerous competitors, with new iterations appearing almost daily, each one promising improvements over the last. If you are not sure which one to choose, or if you would like to compare results from several chatbots – or even to ask your students to do so – then you could turn to Poe, which pulls together OpenAI’s ChatGPT, Anthropic’s Claude, Google’s Gemini, and many more.
Some (though, as of 2024, not yet all) generative AI chatbots have live internet access, so the line between generative AI and search is beginning to blur. Examples include Google’s Gemini, Microsoft’s Copilot, and Perplexity AI. When you come across such a service, you might well ask yourself: is this a generative AI chatbot; or is this a search engine? The answer is yes; and yes. This fusion of generative AI and search would seem to be the way of the future.
Speaking of the future: current trends include a shift away from publicly available LLMs towards more secure enterprise or on-device tools (the latter sometimes labelled small language models, or SLMs) among users with financial means, especially in the Global North; the integration of generative AI into older, first-generation conversational AI interfaces like Amazon’s Alexa and Apple’s Siri; and the intertwined evolution of semi-autonomous personal agents, likely anchored in on-device SLMs, which can converse in everyday language and navigate and control smartphone (or computer) apps (Low, Reference Low2024). In other developments, in February 2024 an intriguing post appeared on X, formerly Twitter, from Logan Kilpatrick, who at the time led developer relations at OpenAI. He wrote: ‘The final form for ChatGPT is not chat :)’ (@OfficialLoganK, 2024). What, then, might it look like?
Given the later announcement of OpenAI’s collaboration with robotics company Figure, one possibility is the emergence of humanoid AI robots, with the founder of Figure, Brett Adcock, describing his aim ‘to bring embodied AI into the world to make a transformative impact on humanity’ (cited in PR Newswire, 2024). A number of other companies are also working in this space. This is potentially quite significant, with some AI researchers advocating a shift away from the traditional emphasis on Cartesian dualism – essentially, the idea that the mind and the body are separable – and towards an emphasis on embodied cognition, arguing that strong AI may only be able to emerge once it has a body through which to experience the world (Paolo et al., Reference Paolo, Gonzalez-Billandon and Kégl2024). So, might this be the frontier where strong AI will one day arise?
We will have to wait and see. In the meantime, let us turn to the implications of today’s technologies for education and assessment, with a particular focus on the teaching and learning of language and literacy.
3. An educational view
Students and teachers have now been experimenting with generative AI like ChatGPT since late 2022, and extensive reports have emerged of promising educational uses (Pegrum, Reference Pegrum2024). Students can, for instance, ask AI chatbots to explain grammar points, contextualise vocabulary, devise revision questions, and take on the role of either a teacher or a learner, depending on the context (see Table 1). As we take a look at some examples, we should bear in mind that when the technology is treated as a simple replacement for teacher input or student output, it is potentially very problematic; but when it is used in a supporting role, it is potentially very valuable.
Table 1. Selected student uses of generative AI

In the most controlled environments, students can engage in individualised learning activities and receive individualised feedback. For instance, my Australian colleague Kate Kerr reports on successfully using Microsoft’s Reading Coach with young learners of English, who can co-create a story using generative AI, read passages aloud, and obtain feedback on the accuracy of their pronunciation and guidance on words they need to practise.
At higher levels, students can make use of GPT-based tutors such as the Khan Academy’s Khanmigo, set up as a Socratic tutor who refuses to give students direct answers to their questions, but rather guides them step-by-step towards finding their own answers. A similar GPT-based tutoring function is seen in Duolingo Max, a premium offering from the language learning app Duolingo, initially available for French and Spanish (Caldwell, Reference Caldwell2024). Students converse with an AI tutor who can, for example, explain grammar points in multiple steps, or engage in target language role-plays and then give feedback on them. Some teachers are also experimenting with having their students interact with historical or fictional figures through platforms like Hello History and Character AI (both multilingual).
Fluid conversational scenarios with human oversight may hold even more promise for language practice. An Argentina-based colleague, Doris Molero, reports on supervising an advanced learner of English who engaged in role-plays with the Pi AI chatbot, which could be asked to take on various personas such as, in one case, a car dealer. In another class, Doris used both ChatGPT and Google’s NotebookLM with an English learner who was tasked with interviewing one of her favourite singers. When the student initially struggled to come up with in-depth interview questions, inspiration was sought from NotebookLM, which can generate questions pertaining to relevant information sources. The student’s homework was to polish up a question set for the next class, where she then role-played a ‘live’ interview with the singer, who was simulated by ChatGPT.
There are numerous tools which can help students to make a start on their tasks. When studying large amounts of material or revising for exams, students could employ software like Mindgrasp (which supports more than 30 languages) to automatically create summaries and take notes from documents or artefacts ranging from PDF files to videos. When English learners are exploring academic literature, they could employ software like Elicit to identify relevant references and compose a first draft of a literature review, which they could then critically edit and supplement with further literature in order to deepen and broaden the analysis. The text below summarises the ‘top 4 papers’ found by the free version of Elicit in response to my question ‘How can language learners benefit from generative AI?’ (updated 16 October 2024):
Generative AI offers significant opportunities for language learners and educators. It can provide personalized practice, feedback, and study plans (Godwin-Jones, Reference Godwin-Jones2024), as well as support Universal Design for Learning to benefit all learners, including English learners and students with disabilities (Evmenova et al., Reference Evmenova, Borup and Shin2024). AI-powered tools can enhance language acquisition through customized learning journeys and dynamic content (Creely, Reference Creely2024). However, challenges exist, including ethical concerns about authenticity and intellectual property (Creely, Reference Creely2024), and limitations in addressing nuanced social and cultural aspects of language use (Godwin-Jones, Reference Godwin-Jones2024). There are also concerns about AI’s potential to standardize language expression and propagate limited cultural narratives (Creely, Reference Creely2024). Despite these challenges, generative AI is reshaping second language education, with numerous AI-powered language learning tools widely available and used globally (Davoodifard & Eskin, Reference Davoodifard and Eskin2024). A balanced approach that leverages AI’s benefits while addressing its drawbacks is recommended for language education in the emerging AI-centric digital landscape (Creely, Reference Creely2024).
While such tools can help students get started, other tools exist to help them improve on what they themselves have started, for example honing their clarity, formality, or tone. Well-known services Grammarly and Wordtune, though offering some translation features, are focused on composing and revising English-language text, but multilingual alternatives exist.
Varied teacher uses of generative AI have also emerged. The most obvious possibilities include creating lesson plans, teaching materials, and assessments and rubrics (see Table 2). Some teachers have gone further by designing their own dedicated learning chatbots, while others have experimented with using generative AI to provide feedback to students, to analyse student data, and even to draft student reports, but it should be noted that there are permission and privacy concerns with the uploading of sensitive data into publicly available systems, which may retain it and incorporate it into their training datasets.
Table 2. Selected teacher uses of generative AI

In a 2023 survey of over 350 teachers of 16 different languages, my colleagues Antonie Alm and Louise Ohashi, based respectively in New Zealand and Japan, found that language teachers were open to using ChatGPT to create the following kinds of resources, ordered here by teachers’ levels of openness towards creating them (see Table 3; note that ‘Conversation’ and ‘Quizzes and tests’ were equally rated). Certainly, all of these uses are possible.
Table 3. Language teachers’ openness to using ChatGPT to create resource types, from highest to lowest openness (source: adapted from Alm & Ohashi, Reference Alm and Ohashi2024)

Let us take a look at some examples of teacher uses of generative AI. Twee is one of the older generative AI tools and is aimed at teachers of English, including English as a foreign, second, or additional language, but could equally be used across a range of other subject areas. Options include automatically generating questions about YouTube videos; generating dialogues or stories at a specified level of the Common European Framework of Reference for Languages (CEFR) and incorporating specified structures and lexis; and building everything from multiple-choice questions to fill-the-gap exercises. A swathe of similar services, each with its own points of emphasis, is now appearing. The multilingual Diffit can automatically build a whole lesson around a given video or text, generating resources and activities at a specified school grade level and in a specified language, including bullet point summaries, vocabulary lists, graphic organisers, and multiple-choice or short answer questions. AI functionality is also being introduced into older platforms, perhaps best exemplified by Padlet (dating back to 2008, when it was known as Wallwisher), which now offers boards created from a series of AI recipes including ‘Lesson plan’, ‘Ideas for class activities’, ‘Assessment polls’, and ‘Rubric’.
Some of the most innovative approaches involve the use of multiple platforms in combination to create materials closely tailored to class needs. An Australian colleague, Sandra Houghton, reports how she developed a lesson on community projects for her English learners by using Magic School to generate lesson stages, ChatGPT to produce a reference list of key functional language, Canva’s Magic Studio to integrate all of this into a handout, and finally Canva’s AI Presentation Maker to create slides signalling lesson stages and reminding students of the key language. In a rather different example, Doris Molero, whom I mentioned earlier, composed an environmentally themed story for her young English learners using ChatGPT, illustrated it with Copilot, compiled the text and images into a book with Book Creator, and then set her students questions using Twee.
In the future, we may also see many language teachers creating their own customised AI chatbots, such as Doris’s Pionia Destiny on Character AI, which her students use for homework conversations on new topics covered in class. Meanwhile, Louise Ohashi demonstrates a self-created GPT chatbot to her tertiary level learners of English in Japan before inviting them to experiment with collaboratively creating customised bots on Poe, focusing on their preferred areas of language practice.
Creativity and critique can and should go hand-in-hand. Borrowing an activity suggestion from the Generative AI idea pack for English language teachers (CUP [Cambridge University Press], 2024), I entered the prompt ‘Create a poster of Australian people’ into Copilot to see how my own nationality might be represented; one example is reproduced below (see Fig. 3). This activity naturally leads to analysis and critique of cultural representations, and potentially to the building of intercultural literacy. It can also lend itself to creativity, and potentially to the building of prompt literacy, if students are invited to craft their own detailed prompts to generate images that capture their perspectives and, ideally, reflect a somewhat broader representation of identities and lifestyles than what we see here.

Figure 3. A poster of Australian people. DALL-E 3 image created by Mark Pegrum (2024) via Microsoft Copilot.
Generative AI has implications not only for learning, but for assessment. One possible approach is to try to catch students out if they overuse generative AI support, as plagiarism detection companies aim to do. Although these companies claim high detection rates for AI-generated texts, many researchers remain unconvinced that AI detectors are reliable (Ardito, Reference Ardito2024; Sadasivan et al., Reference Sadasivan, Kumar, Balasubramanian, Wang and Feizi2024; Weber-Wulff et al., Reference Weber-Wulff, Anohina-Naumeca, Bjelobaba, Foltýnek, Guerrero-Dib, Popoola, Šigut and Waddington2023). There is, moreover, an unavoidable lack of transparency in AI detection:
Although text matching software also suffers from false positives and false negatives (Foltýnek et al., Reference Foltýnek, Dlabolová, Anohina-Naumeca, Razı, Kravjar, Kamzola, Guerrero-Dib, Çelik and Weber-Wulff2020), at least it is possible to provide evidence of potential misconduct. In the case of the detection tools for AI-generated text, this is not the case. (Weber-Wulff et al., Reference Weber-Wulff, Anohina-Naumeca, Bjelobaba, Foltýnek, Guerrero-Dib, Popoola, Šigut and Waddington2023, p. 27)
More concerning still is research suggesting that the approaches taken by AI detectors, which rely on factors like perplexity and burstiness (essentially, measures of the unpredictability of a text), are inherently biased against those who use language in more constrained ways. This includes not only non-native speakers (Liang et al., Reference Liang, Yuksekgonul, Mao, Wu and Zou2023; note that it may be possible to correct for such bias with customised detectors, per Jiang et al., Reference Jiang, Hao, Fauss and Li2024) but may also include those with autism spectrum disorder (ASD), as flagged up in a white paper from Anthology (2023), the company behind the Blackboard Learning Management System (LMS). This has enormous ramifications in terms of diversity, equity, and inclusion.
On the other hand, as pointed out in a recent UNESCO guide, generative AI has the potential, albeit still largely untested at scale, to benefit learners with specific needs, including through AI-powered accessibility tools (e.g. for learners with hearing or visual impairments) and as a ‘[g]enerative amplifier for marginalized users’ (e.g. learners from minority linguistic or cultural backgrounds) (Miao & Holmes, Reference Miao and Holmes2023, pp. 34–35). There is an apparent contradiction here. A moment ago, we saw that generative AI could serve to exclude diverse students; here, we read that it could help to include diverse students. To me, what this says is that much more research is needed on exactly how generative AI can be used to support diverse students in their learning and assessments.
In the era of generative AI, a better approach to both learning and assessment for all students may be to set more tasks at the higher levels of Bloom’s Revised Taxonomy, something which has indeed been discussed for many years in the context of developing students’ higher-order thinking skills. This might mean students critiquing a text generated by an AI chatbot and seeking to improve on it; or asking the chatbot for feedback on their own work and then offering feedback on the feedback, indicating which elements they would act on, which they would not, and why; or engaging the chatbot in iterative co-creation of artefacts contextualised by the students to address real world needs or challenges. In sum, the most promising educational approaches are likely to be those where students demonstrate their ability to work effectively in human AI-partnerships.
In the Microsoft new future of work report 2023, it is suggested that there are many different formats and levels for collaboration between humans and AI, but that human-centred complementarity should be the goal (Butler et al., Reference Butler, Jaffe, Baym, Czerwinski, Iqbal, Nowak, Rintel, Sellen, Vorvoreanu, Hecht, B. and Teevan2023, p. 15). This fits neatly with an idea proposed by Cornell law professor Frank Pasquale who, well before the current generative AI explosion, had already suggested that we should be focusing less on AI and more on IA, by which he means intelligence augmentation – that is, machines augmenting human intelligence (Pasquale, Reference Pasquale2020). More recently, a similar idea has been proposed by University of Pennsylvania researcher Ethan Mollick under the name of co-intelligence (Mollick, Reference Mollick2024a). Whether we call it complementarity, or augmentation, or co-intelligence, the concept is broadly similar: the future of both study and work is all about humans and AI collaborating.
4. A societal view
We said earlier that, overall, generative AI could contribute to making education better, or it could contribute to making it worse. It brings with it a raft of issues, some of direct pedagogical relevance, and some of broader societal relevance. Let us take a quick look at four key sets of issues.
First, there are issues of accuracy and reliability. On its homepage, ChatGPT offers this casually worded warning: ‘ChatGPT can make mistakes. Check important info’. One of the greatest concerns with generative AI is the erroneous responses it can produce; as mentioned earlier, these are known as hallucinations in the narrow sense of the term. They extend from inventing facts to inventing research studies and references (Sharples, Reference Sharples2023). Some hallucinations, moreover, may have real world consequences that end up in law courts. Here are just three news headlines regarding false accusations made by AI in cases spread across Australia, the USA, and Germany:
Hepburn mayor may sue OpenAI for defamation over false ChatGPT claims – ABC News, Australia (Mayers, Reference Mayers2023)
OpenAI sued for defamation after ChatGPT generates fake complaint accusing man of embezzlement – Forbes, USA (Ray, Reference Ray2023)
Microsoft’s AI falsely accuses reporter of crimes – MSN, USA (Landes, Reference Landes2024)
There is also some danger of hallucinations and misinformation accumulating. If, as is likely, generative AI increasingly comes to be trained on the outputs of other generative AI, this may lead over time to degraded results – and if this phenomenon becomes widespread enough, it could result in model collapse, effectively, the collapse of generative AI as we know it (Marr, Reference Marr2024; Shumailov et al., Reference Shumailov, Shumaylov, Zhao, Papernot, Anderson and Gal2024). Of course, aside from unintentionally false information, or misinformation, there is the matter of intentionally false information, or disinformation. One looming dilemma is how best to regulate, and educate about, today’s proliferating deepfakes, where AI is used to generate fake images, videos, or audio recordings, often of well-known people, that misrepresent their words or actions.
Second, there are issues of standardisation and homogenisation, including of our language. There are copious online lists of overused ChatGPT words such as delve, tapestry, landscape, and realm, and phrases such as dive into, based on the information provided, navigating the landscape, and a testament to (Dan, 2024). These may be useful for teachers as telltale signs of student use of generative AI, while students could be asked to generate such lists themselves as an awareness-raising exercise. Yet it is not just students’ writing that is affected; so too is the writing of academics. Following an approach suggested by Swinburne University of Technology lecturer Jeremy Nguyen in a widely read post on X (@JeremyNguyenPhD, 2024), if we conduct a search for occurrences of delve (the first verb in Dan’s list above) in papers in the PubMed academic database, we find a jump from 510 occurrences in 2021 (the year before the release of ChatGPT), to 629 in 2022 (the year of its release on 30 November), to 2,872 in 2023 (the first full year of its availability), and to 9,193 as of mid-November 2024. While correlation does not prove causation, this is certainly suggestive of the influence of ChatGPT on academic writing. Moreover, there is likely to be a feedback loop here. As we, as readers and researchers, start to come across particular terms and formulations more often, we may subconsciously begin using them more often ourselves. In this way, AI could make inroads into homogenising our language usage.
My next example might initially sound like the polar opposite of homogenisation, but I would argue that it is a different, and potentially more acute, manifestation of a similar underlying process of standardisation, not so much of language but of learning itself. I believe that when we are talking about generative AI, educators should avoid the term ‘personalisation’, which seems to have leaked into our discourse directly from technology companies’ marketing slogans (for related recent commentary, see Furze, Reference Furze2024). In general, AI is anything but personal. It limits our personal connection with human interlocutors. More importantly, it often limits our personal agency by only presenting us with restricted sets of recommendations and options which are predetermined by hidden and generally unknowable ‘black box’ algorithms, to borrow Pasquale’s (Reference Pasquale2015) term. Perhaps most importantly of all, it limits our personal integrity by treating us not as whole people but as disaggregated and reaggregated datasets which can be collected, manipulated, and, not infrequently, monetised. Thus, it seems preferable to avoid the conceptual slippage occasioned by the humanistic connotations of ‘personalisation’; at best, I would say, we can call this phenomenon ‘individualisation’, but with the recognition that it is interwoven in complex ways with standardisation. We should also remember that although there is a place in education for individualised learning, notably in a supporting self-study role, it is potentially problematic in the classroom, where it may undercut decades of pedagogical trends towards collaborative, social constructivist learning that involves people interacting with other people.
Third, there are issues of bias and discrimination. You may recall a controversy from late 2020 when the high-profile computer scientist Timnit Gebru left Google due to a conflict over a then unpublished, co-authored paper about AI (Hao, Reference Hao2020; Harris, Reference Harris2023). In this paper, published the following year, the authors warn about the dangers of AI trained on large internet datasets: ‘In accepting large amounts of web text as “representative” of “all” of humanity we risk perpetuating dominant viewpoints, increasing power imbalances, and further reifying inequality’ (Bender et al., Reference Bender, Gebru, McMillan-Major and Shmitchell2021, p. 614). The paper’s clarion call has continued to echo through a swathe of subsequent posts, presentations, and publications highlighting the multifarious forms of bias – pertaining to (dis)ability, gender, sexuality, race, language, socioeconomic status, education, and more, not to mention intersectional bias – which are present in training datasets. Notwithstanding recent efforts by technology companies to diversify the output of generative AI, underlying biases can and do continue to flow through into the texts and images generated, sometimes in obvious ways, and sometimes – perhaps more dangerously – in rather subtle ways (Cao et al., Reference Cao, Zhou, Lee, Cabello, Chen and Hershcovich2023; Cooper & Tang, Reference Cooper and Tang2024; Walker Rettberg, Reference Walker Rettberg2024).
Last, but certainly not least, there is the issue of environmental impact. All digital tools are energy hungry and environmentally destructive, but this is particularly true of the training and operation of AI, as has been well documented at least since the publication of Gebru’s co-authored paper (Bender et al., Reference Bender, Gebru, McMillan-Major and Shmitchell2021; Crawford, Reference Crawford2024; Saul et al., Reference Saul, Nicoletti, Rai, Bass, King and Duggan2024; Verma & Tan, Reference Verma and Tan2024). To borrow just two examples from Vicky Saumell’s opening plenary at the 2024 International Association of Teachers of English as a Foreign Language (IATEFL) Conference in the UK: creating one AI-generated image can use as much electricity as fully charging your smartphone (AI for Education, 2023); and for somewhere between 5–50 responses, depending on timing and location, the (old) GPT-3 needed to ‘drink’ half a litre of water (Li et al., Reference Li, Yang, Islam and Ren2023). As Vicky herself pointed out, whenever we are refining our prompts over and over again, we should be considering the water consumption (Saumell, Reference Saumell2024).
As John Naughton wrote recently in The Guardian: ‘AI technology is an environmental disaster in the making; the planet cannot be paved with datacentres’ (Naughton, Reference Naughton2024). Gebru and her colleagues use the term environmental racism to highlight the fact that the negative climate and environmental costs of AI development and deployment are disproportionately borne by those in the Global South, alongside marginalised populations in the Global North, who benefit the least from these kinds of technologies (Bender et al., Reference Bender, Gebru, McMillan-Major and Shmitchell2021, pp. 612–613).
In coming years, we will hear increasing mention of movements like green it and computing within limits, focused on more environmentally sustainable approaches to our technologies (Nardi et al., Reference Nardi, Tomlinson, Patterson, Chen, Pargman, Raghavan and Penzenstadler2018). Maybe it is also time, as Neil Selwyn has suggested, to start thinking about ed-tech within limits:
we need to anticipate the ongoing environmental degradation of the planet as radically upending existing assumptions about the continued use of digital technologies in education. (Selwyn, Reference Ng, Leung, Chu and Qiao2021, p. 501, italics in original)
If anything, this has become an even more pressing issue in the era of generative AI. Taken seriously, it may mean switching off our educational technologies at least some of the time.
We are beginning to see educational initiatives addressing the societal challenges of AI. In 2023, for example, the Australian framework for generative artificial intelligence in schools identified ‘Human and Social Wellbeing’ as its second of six core areas, with a focus on wellbeing, diversity, and human rights (Department of Education [Australia], 2023). The next year, the aforementioned Generative AI idea pack for English language teachers included an activity called ‘How sustainable is AI?’, designed to raise students’ critical awareness of economic, social, and environmental issues (CUP [Cambridge University Press], 2024). Such educational initiatives represent the first tiny steps towards addressing very large problems.
5. Conclusion: The need for AI literacy
Hopefully it is evident by now that in order to ensure AI supports rather than detracts from the ongoing evolution of education, we as educators need to develop our AI literacy. Ultimately, we also need to help our students develop their AI literacy to prepare them for their future studies, careers, and lives, all of which will be infused with AI. Time does not permit me to go into great detail here, so I will wrap up with a few brief comments.
AI literacy is just one of a web of interrelated literacies, ranging from search (and now prompt) literacy through to ethical and critical literacy (Pegrum et al., Reference Pegrum, Hockly and Dudeney2022). This is abundantly clear in early attempts to define AI literacy. Prior to the recent generative AI explosion, the best-known conceptualisations included Long and Magerko’s (Reference Long and Magerko2020) set of three competencies: critically evaluate AI, communicate and collaborate with AI, and use AI; and Ng et al.’s (Reference Ng, Leung, Chu and Qiao2021) set of four aspects: know and understand AI, apply AI, evaluate and create AI, and AI ethics. In the post-ChatGPT era, the latter has been subsumed into a newer pedagogical framework highlighting the affective, behavioural, cognitive, and ethical dimensions of learning about AI (Ng et al., Reference Ng, Wu, Leung, Chiu and Chu2024).
My own ongoing work, conducted with my colleagues Ekaterina Tour from Monash University and Shem Macdonald from La Trobe University, focuses on the application of AI literacy to language learning in particular (Tour et al., Reference Tour, Pegrum, Macdonald, McCallum and Tafazoliin press). Our model delineates four domains: the operational, contextual, critical, and creative. This is a space to watch over the next two to three years as we will see a rush of presentations and papers attempting to define and operationalise AI literacy for the classroom, including for specific areas like language teaching and learning.
I will close with an image I asked DALL-E 3 to create for me, representing AI literacy. I am sure it will not take you long to spot something out of place here in the form of an extra letter ‘E’ in ‘LITERACY’ (see Fig. 4). In brief, there is no danger of this technology replacing teachers or textbooks any time soon, much less revolutionising entire education systems. Nevertheless, in a supporting role, it can be extremely valuable. As educators, we urgently need to develop our AI literacy, both to ensure that we are integrating AI effectively as a support for the ongoing evolution of education, and to ensure that we are well-placed to help our students begin developing their own AI literacy.

Figure 4. An image of AI literacy. DALL-E 3 image created by Mark Pegrum (2024) via Microsoft Copilot. Circle added by author.
Mark Pegrum lectures on digital learning in the Graduate School of Education at The University of Western Australia in Perth, where he is also the Deputy Head of School (International). His current research focuses on emerging technologies, including generative artificial intelligence (AI); digital literacies, including attentional literacy and AI literacy; and the forms digital learning takes in diverse contexts across the Global North and South. His books include: Mobile learning: Languages, literacies and cultures (Palgrave Macmillan, 2014); Mobile lenses on learning: Languages and literacies on the move (Springer, 2019); and Digital literacies (2nd ed., co-authored with Nicky Hockly and Gavin Dudeney, Routledge, 2022). Further details can be found on his website at https://markpegrum.com/about-mark-pegrum/biodata/.